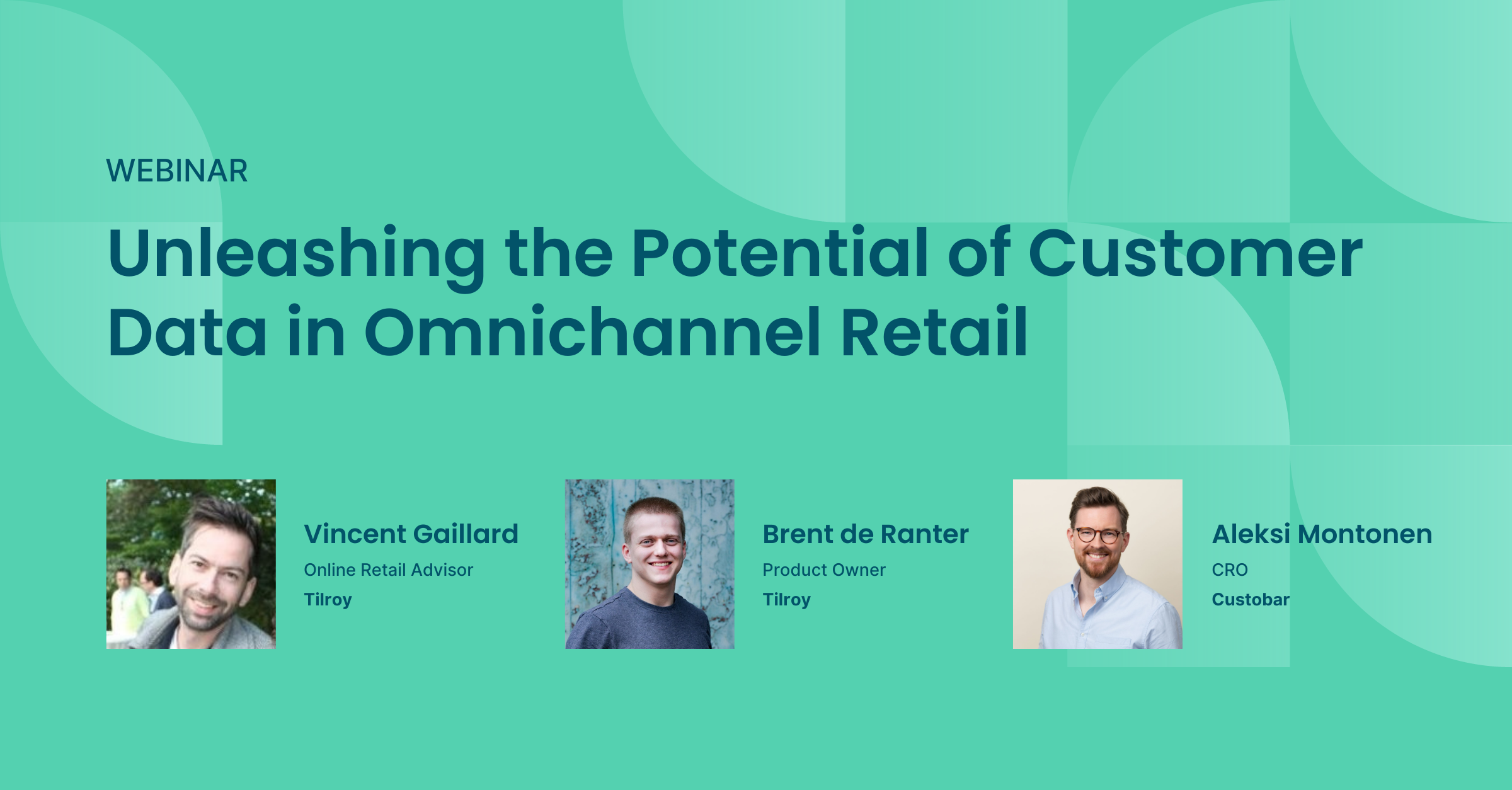
Unleashing the potential of customer data in omnichannel retail
Watch the webinar recording with Vincent Gaillard and Brent de Ranter from Tilroy and Aleksi Montonen from Custobar.
Marketing automation cost-efficiently increases online sales, engages customers, and reduces churn.
Filter with tags
Marketing automation cost-efficiently increases online sales, engages customers, and reduces churn.
After Tatu Kuivalahti has led Custobar successfully for a decade, Laura Ek is taking over the CEO role at Custobar
We're pleased to announce several significant updates to the Custobar platform.
In February 2024, Google and others are implementing many new requirements for bulk email senders.
Email domain providers Google and Yahoo have both announced that starting 1st February 2024, stricter requirements on senders of bulk email will come into effect.
European data protection law has brought about a profound transformation in how customer data is handled.
Black Friday is now behind us, and the holiday sales season is in full swing. We are excited to announce that the November 2023 product updates, including Periodic Data Updates, for Custobar are now live!
Turning passive customers into active ones is an essential strategy for any business.